
I’m here to help you avoid my mistakes early in my career. At some point in time, we were all told to learn stats. But just learning stats is not sufficient. Interpretation and context are also critical. Consider the scenario below.
The image below shows the annual salary of 7 employees in an organization.
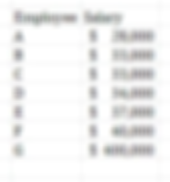
If you are to answer the question of what is the average salary of this company? Are your thoughts on calculating the mean right? But that would give the wrong answer. I will show you why.

If we were to calculate the average of all salaries, we would have an average of $86,429, which is not a reflection of the actual data.

If you skim through the salaries, $86,429 is a far-fetch as no team member except “G”, an outlier, earns close to that amount. The median, in this case, is a better reflection of what the average salary looks like.
Note that the $86,429 can’t be said to be wrong. This is one way to lie with data. Say they were my team member, and I’m the employer “G”. The labour union is protesting that my employees are being underpaid, and I’m being interviewed by one of the popular news channels.
If I say the average salary in my organization is $86,429, their protest is unjustified. The statement in literal terms is not wrong, but I just lied with data because, due to the single outlier of $400,000, the average is no longer an accurate reflection of the data.
Distribution of the data is essential to the interpretation of your statistical analysis. I wrote about how the distribution data dictate how I treat missing values here.

Let’s dive into descriptive statistics. So what is descriptive statistics?

Investopedia defined descriptive statistics as brief informational coefficients that summarize a given data set, which can represent the entire population or a sample of a population.
Before we go on, let’s break down the difference between a sample and a population.
Consider this scenario. As a result of the food inflation, you are surveying the prices of food across the country.
Your population size is everyone selling food items in all markets (both major and minor). Seem like an unrealistic thing to achieve. Going to all markets and meeting every vendor asking about the prices of food items requires a hefty amount of resources. That’s where your sample size comes in.
The sample size is just a subset of your population. Instead of going to all the markets in the country for your survey, you go to selected markets and vendors, not necessarily all vendors, and then use the data from that subset to make inferences on the entire population. For instance, I can go to selected markets here in Abuja and then use that data to say this is the average price of rice in Abuja. I don’t need to visit every nook and cranny of Abuja.
Enough deviation. Let’s get back on track.

Descriptive statistics can be broadly grouped into the following:
Measures of frequency — tells you how often something occurs, e.g. count, percentage and frequency.
Measures of central tendency — captures the central aspect of a data, e.g. mean, median and mode.
Measures of dispersions — captures how spread out or dispersed the data is, e.g. range, variance and standard deviation.
Measures of Central Tendency

This summary measure aims to summarize all the data in a collection by using a single value corresponding to the middle or centre of the distribution. They include mode, mean and median.
The mean refers to the average of the data. The median of a set of ordered observations is a middle number that divides the data into two parts, while the mode is the most frequent number.
If you notice extreme observations (outliers) in your data, then a median is a better summary statistic to report than a mean.
Also, comparing the mean with the median tells you a bit about the distribution of your data. If Mean > Median, the data is skewed to the right (i.e. positively skewed). The quick hack to understand skewness is the length of the tail. Here, a long tail is on the right side compared to the left.
If Mean < Median, the data is skewed to the left (i.e. negatively skewed). There is a long tail on the left side compared to the right.

Measures of Dispersion
Earlier, we said, this set of measures captures how spread out or dispersed the data is. To better understand this, consider the salaries of two different organizations.
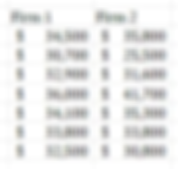
Let’s plot the salaries.

Notice how the salaries in firm 1 are more closely knitted than in firm 2, even though the mean and median are equal. That’s what the measure of dispersion helps us understand, i.e. how spread out the values are.

How can this spread difference be converted into a descriptive statistic that means something?

Calculating the data range, which is only the difference between the data’s maximum and minimum values, is one method for doing this.

Firm 1’s highest and minimum salaries are $36,000 and $30,700, respectively, giving us a range of $5,300. The range of wages in Firm 2 is also $16,200. A wider range denotes more data spread or dispersion.
One more comparable measure follows the range measure of dispersion. I’m talking about the inter-quartile range or IQR.

This describes the central 50% of the data, leaving the remaining 25% to the right and 25% to the left. A number is considered in the first quartile if 25% of the observations fall within this range. A third quartile is a number that 75% of the observations must be less than or equal to.

Incidentally, the second quartile corresponds to the median. The zeroth quartile is the lowest value in the range. The fourth quartile represents the highest value in the range. The interquartile range or IQR is the third quartile, less the first quartile.

Why do we prefer the interquartile range over the range measure?

Suppose you are working with sample data and trying to make inferences about the sample data’s population. In that case, A sample’s range is not always representative of the population it originates from. On the other hand, the interquartile range of a sample is representative of the population’s interquartile range.
Let’s look at a box plot, which is an excellent tool for displaying various descriptive statistics.
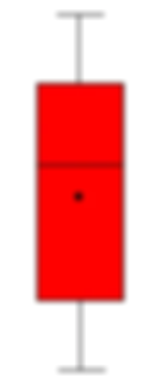
Box plots are sometimes known as box and whisker plots. The two whiskers at the top and bottom indicate your data’s maximum and minimum values, respectively. The rectangle between the first and third quartiles is your interquartile range. The dot represents the mean, and the horizontal line within the box represents the median. As a result, you get a practical data summary in a single graphic.

A box plot is handy when making comparisons—for example, the earnings of both male and female football stars.

From the box plot, we can deduce that football’s highest-paid female player makes almost as much as the sport’s highest-paid male player. However, the spread or dispersion of female stars’ earnings is greater than that of male stars. The range, which is the range between the maximum and minimum, serves as proof. Additionally, there is the rectangle box’s height and the interquartile range. Female stars’ mean earnings are higher than their median earnings is another intriguing finding. However, the mean income for the male stars is lower than the median income. Implying that, compared to male stars, the earnings distribution for female stars tends to be significantly more right-skewed. A few female football players with very high salaries contribute to the total mean exceeding the median income.
We have covered two measures of dispersion, the range and the interquartile range. Let’s see the difference with the typical standard deviation. Consider the salary scenario for the seven employees below.

The spread or dispersion of data is described by the difference between some high values and some low values using the range and interquartile range metrics. The range, for instance, is the difference between a data’s maximum value and minimum value.

The difference between a high number, the third quartile, and a low value, the first quartile, is the interquartile range.

However, the standard deviation first determines the data’s mean.

Then calculates the variations between each data point and the mean.

It then combines these differences to give the standard deviation measure. N in this formula is the total number of observations. The standard deviation formula sums the square of differences, divides it by N, and then takes the square root of the result.

Now we have calculated the standard deviation. But the number is useless without interpretation. For this, consider the salary data below.
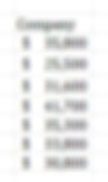
The standard deviation is calculated as follows in Excel.

What does the $5,013 mean?

To understand this, we need to recall the rule of thumb. It says that
approximately 68% of data lie within one standard deviation, and approximately 95% lie within 2 standard deviations from the mean
Therefore, our data shows that 68% of salaries were $33,500 ± $5,013 (1 standard deviation away from the mean). 95% of salaries were $33,500 ± (2 * $5,013), i.e. two standard deviations from the mean.
Variance, another measure of dispersion, is the square of your standard deviation.
That marks the end of this piece. Adios
